Working with R and GeoDa:
Spatial Autocorrelation
CalEniviroScreen 4.0 data was used in this project to demonstrate the implementation of spatial autocorrelation in R and GeoDa for counties in California. Specifically, the unemployment rate was utilized to map the unemployment rates per county, queen and rook nearest neighbors, total distance, and the queen contiguity of most and least connected neighbors.
Working with R and GeoDa:
Multiple Regression Analysis
This project aims to demonstrate multiple regression, geographically weighted regression, and spatial autocorrelation using two datasets.
​
The first part of this project uses U.S. Covid-19 county-level data to examine death rates. Spatial weights were applied and mapped with Moran's I and Local Indicator Spatial Autocorrelation. Finally, a multiple regression model was applied to the data revealing the statistical significance of the variables contributing to Covid-19 deaths.
​
The second part of this project uses crime data from St. Louis, MO, to examine the impacts of police expenditure and the local deprivation index on the homicide rate within the study area. After generating the multiple regression model, the Local R-squared and coefficients are mapped to show the impacts of the independent variables on the homicide rate.
​
​
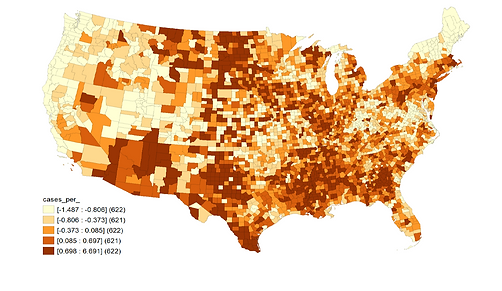